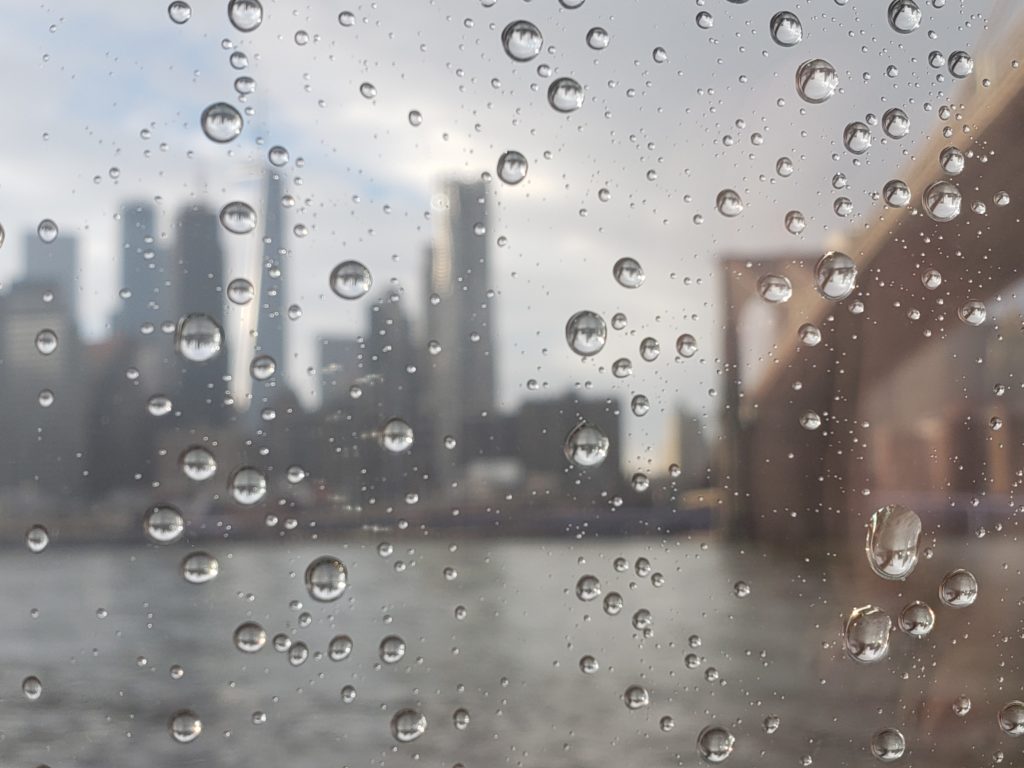
在美国新冠疫情爆发之前,我偶尔也在家工作。因为我平时通勤的时间很长(单程90分钟),所以每次在家工作的时候,都觉得偷得浮生半日闲,可以用省下的时间读读书,做做瑜伽,或者睡个懒觉。我从来没想过在家工作会成为新常态,也没有做好在家打持久战的准备。同样没有做好准备的是我的猫,Maru,它估计在想“铲屎官怎么还不出门给我赚钱?她居然敢坐在我睡觉的御座上?能不能不要在我打盹的时候骚扰我啊!!!”
在美国新冠疫情爆发之前,我偶尔也在家工作。因为我平时通勤的时间很长(单程90分钟),所以每次在家工作的时候,都觉得偷得浮生半日闲,可以用省下的时间读读书,做做瑜伽,或者睡个懒觉。我从来没想过在家工作会成为新常态,也没有做好在家打持久战的准备。同样没有做好准备的是我的猫,Maru,它估计在想“铲屎官怎么还不出门给我赚钱?她居然敢坐在我睡觉的御座上?能不能不要在我打盹的时候骚扰我啊!!!”
In the pre-COVID19 era, I used to work from home occasionally and treated it as a break from my long daily commute (90 min one-way). I spent the saved time reading, exercising, or sleeping. I never thought that working from home would be the new norm and I was not prepared for a seamless work & life, nor was my cat Maru – he was probably thinking “why is this hooman being not hunting outside? why is she still sitting on my sleeping chair? and stop disturbing my day-time nap!!!”
In the previous post, I talked about using MapReduce and Spark for distributed model training. In this post, I will talk about parameter server and how it is used in distributed model training.
In the previous post, I introduced challenges in machine learning systems with big data and complex models. In this post, I will discuss distributed systems in the era of big data.
Let’s start with linear regression. Using established libraries such as scikit-learn, it is almost trivial to train a linear regression model. We can easily run the model training with a few hundred Megabytes of data on our laptop with a build-in CPU.
Now let’s think big.
In business management, product lifecycle is broken into 4 stages with the distinct pattern of sales over time: introduction, growth, mature, and decline. In the diagram below, I adapt the classic product lifecycle curve to show the engineering load over time in machine learning (ML): from model development to maintenance. Managing and coordinating different stages in ML lifecycle presents pressing challenges for ML practitioners.
The more I work on building end-to-end machine learning (ML) pipelines, the more I realize the importance of system design and infrastructure. ML shares many concerns with traditional software development, and poses new challenges to system design.
It’s been 3 months since I started my new position as a Machine Learning Engineer (MLE) at Spotify. What I like most about this position is that I get to participate in building an end-to-end pipeline, including ideation and experiment, data engineering, machine learning modeling, model serving, online A/B test, monitoring, and many more.